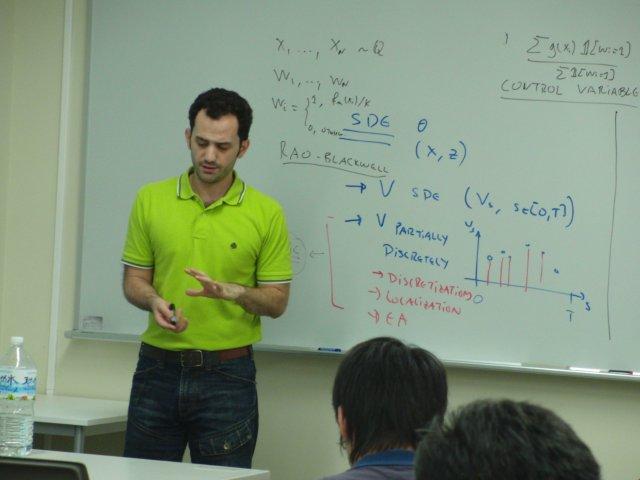
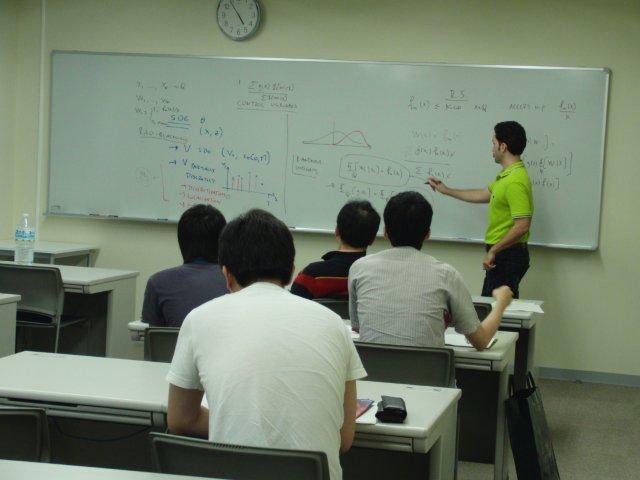


Part Ia: brief introduction to SDEs
(Ito lemma, linear SDEs, generator)
Part Ib: Statistical inference for SDEs
(observation schemes, probabilistic inference, approaches in the literature)
Part II: Statistical inference for the fully observed case
(quadratic variation, Girsanov's theorem for likelihood inference)
Part I and II would be covered in at most 2 hours.Part II is essential
for what follows
Part III: discrete-time dynamics of diffusions and discrete-time likelihood
(discretization schemes, local linearization, pseudo-likelihood, exact
simulation of diffusions)
Part III should be about 1.5 hour.
Part IV: Monte Carlo inference for discretely observed diffusions with
known diffusivity
(missing data and a formal data augmentation, data augmentation for diffusions,
simulation of diffusion bridges, MCMC for parameter estimation)
Part IV should be about 1.5 hour.
Part V: MCMC for general reducible diffusions
(Lamperti transformation and reducible diffusions, lack of convergence of basic MCMC algorithms, transformations of diffusion paths and efficient MCMC)
Part V should be about 1 hour.
Part VI: MCMC for irreducible partially observed multivariate diffusions
(Durham and Gallant approach, general diffusion bridges, path transformations,
unobserved components)
Part VI should be about an 1 hour.
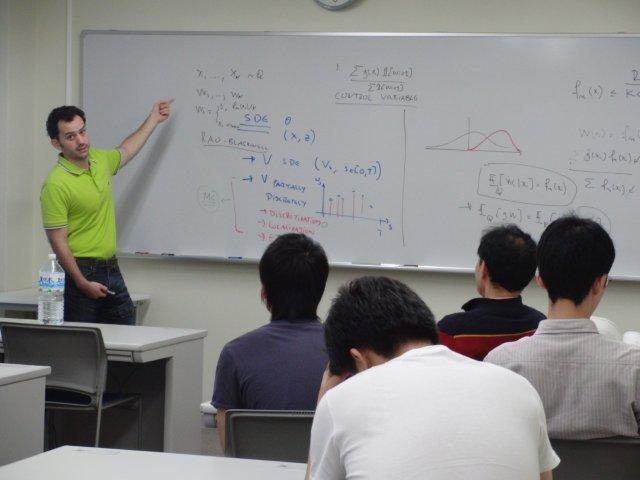
Copyright c 2010 JST/CREST コハツ・チーム
□2010.6.23 - 7.7,16:20-18:30開催 |
Monte Carlo Likelihood-based Inference for Diffusions (モンテ・カルロによる尤度に基づいた拡散過程の推測) |
講演者:Prof. Dr. Omiros PAPASPILIOPOULOS, Asst. Professor in the Dept. of
Economics and Business and Ramon y Cajal Research Fellow at the Universitat Pompeu Fabra
|